An integrated, scalable and reproducible single cell RNA-Seq workflow
The last decade has witnessed a paradigm shift in the way various layers of omics data are being generated and analysed. It is now possible to interrogate every cell of an organism in order to decipher the important biological processes that occur within. At such unprecedented resolutions, we are able to obtain more realistic insights into the composition, dynamics and regulatory mechanisms of cell states in development and disease. One aspect of single cell research that has gained much popularity is single cell RNA-Sequencing (scRNA-Seq), which has emerged as a ground-breaking technology that has greatly enhanced our understanding of the complexity of gene expression at a microscopic resolution. Given the hype, it is anticipated that in the next 5-10 years, the wider research community will be routinely employing this powerful and revolutionary technology as a laboratory staple. This is likely to create an exponential growth of scRNA-Seq data, leading to the big-data problem, a preview of which is the Human Cell Atlas (HCA) project. The aim of the HCA consortium is to create an extensive reference map of all human cells as a basis for understanding human health and diagnosing, monitoring, and treating disease. More than 1,000 researchers have joined this major initiative, some of whom have already catalogued cell types and subtypes from cord blood, bone marrow, spleen and lymph nodes in humans and mice. This has resulted in publicly available datasets of various sizes, ranging from several gigabases to several terabytes, and these numbers are only expected to rise as more data is generated over the next few years.
Given that scRNA-Seq experiments survey thousands of cells at a time, the sizes of the resulting gene expression matrices are already considerably higher compared to bulk RNA-Seq. This is posing serious computational challenges on the data analysis front, given the increase in volume of scRNA-Seq data.
The project involves creation of end-to-end bioinformatics pipelines that are able to pre-process large volumes of raw scRNA-Seq data, post-process and visualise the output in a computationally efficient manner (draft workflow and code available to kick start)
- HPC: adapt this workflow on Pawsey HPC and Cloud
- Embed ML for self organisation maps
- Embed Nextflow architecture for scalability and contanerise for the ease of deployment and reproducibility of these pipelines, a task that can easily be achieved via the use of containers such as Docker, Singularity and Shifter
- Benchmarking the workflow in terms of time and resources used across HPC and Cloud infrastructures
Client
Contact: Assoc. Prof. Parwinder Kaur
Phone: 08 6488 7120
Email: [email protected]
Preferred contact: Email
Location: Crawley
IP Exploitation Model
The IP exploitation model requested by the Client is: Creative Commons (open source) http://creativecommons.org.au/
Department of Computer Science & Software Engineering
The University of Western Australia
Last modified: 22 June 2020
Modified By: Michael Wise
|
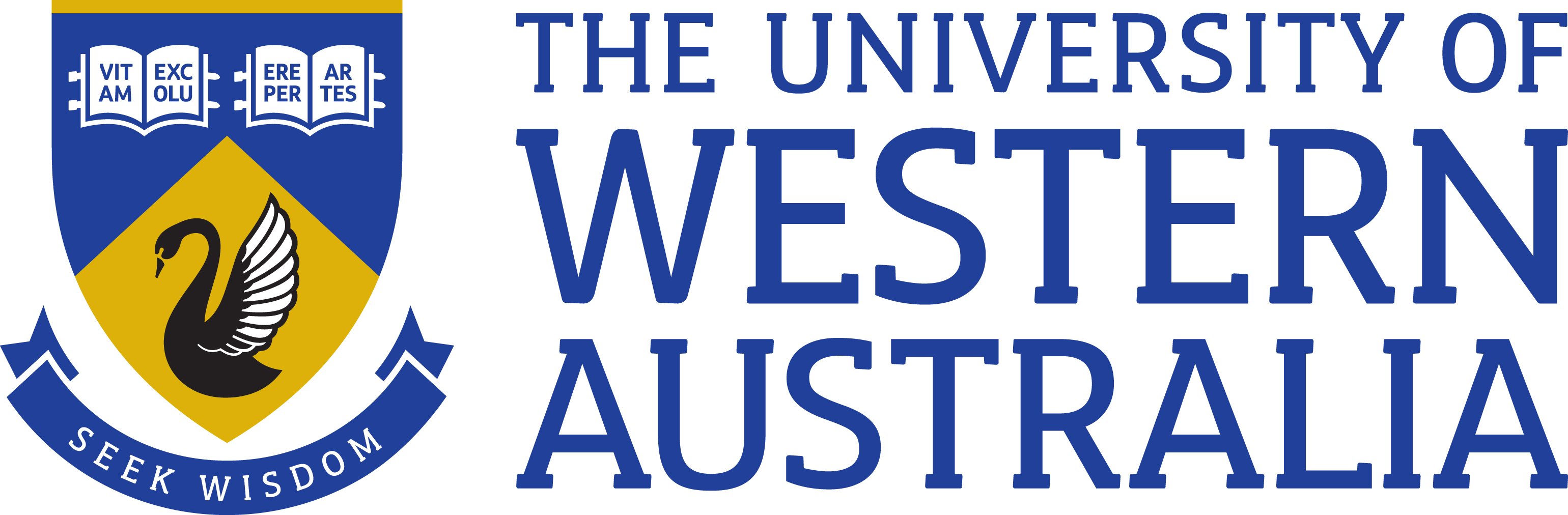 |